18 min read
The Ultimate Guide to Data Products
Written by: Micah Horner, Product Marketing Manager, TimeXtender - December 14, 2023
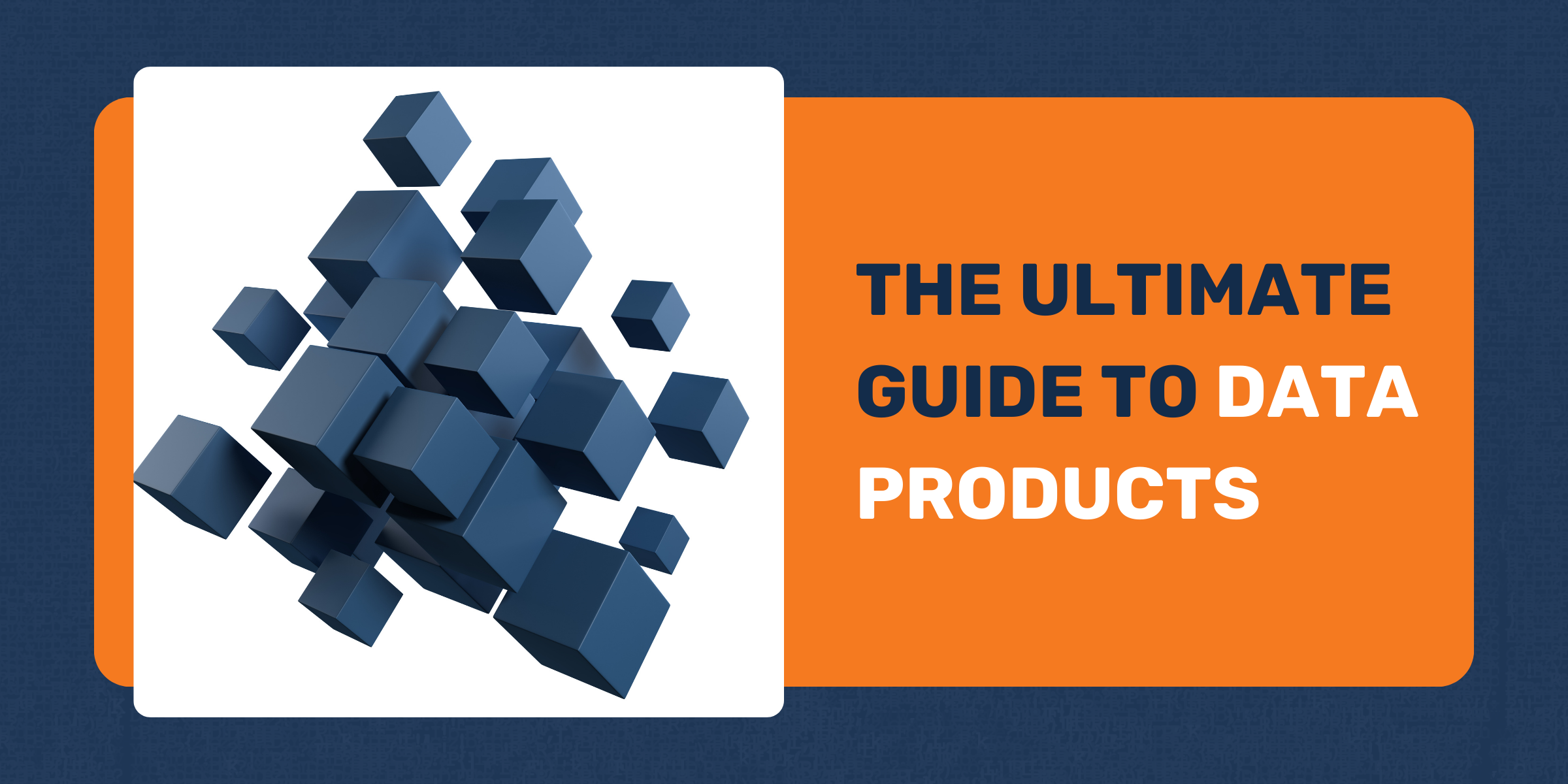
The growing importance of data products in today’s business world marks a significant shift in how data is utilized. Companies across various sectors are harnessing data products to transform raw data into valuable insights, driving smarter decision-making and innovative solutions. This surge in data product use stems from the increasing volumes of data generated daily and the need to convert this data into actionable intelligence. Data products refine and structure this raw data, making it not just comprehensible, but a strategic asset for businesses.
This rise in data products is propelled by advancements in data processing and analytics technology. With more robust computing capabilities and sophisticated data integration tools, managing and interpreting large datasets has become more accessible. The ability to swiftly process and analyze data at scale has turned data products into indispensable tools for organizations, providing clarity and direction amidst a sea of information.
By offering structured, actionable insights, data products empower organizations to respond swiftly to market trends, customer needs, and internal process improvements. They are at the heart of a data-empowered approach, where informed decisions lead to stronger business outcomes and competitive advantage.
What are Data Products?
A data product is essentially a data asset that is refined and structured in such a way that it is ready for use by end users or applications. It has been processed, organized, and presented with a specific purpose or use case in mind.
Data products all share some common characteristics:
-
Structured and Processed: Unlike raw data, data products are organized and formatted for easy consumption. They have been cleansed, standardized, and transformed to ensure accuracy and usability.
-
Purpose-Driven and Outcome-Focused: Data products are crafted with a clear objective, often aimed at solving specific business problems or enhancing decision-making capabilities.
-
Accessible and Usable: A key aspect of data products is their ease of use. They are designed to be easily accessed and interpreted by the intended users, often through user-friendly interfaces.
-
Quality and Governance: Data products are governed by quality standards and policies to ensure they are reliable, secure, and compliant with relevant regulations.
-
Value Creation: The ultimate aim of a data product is to create value – whether that's through improving operational efficiency, enhancing customer experiences, or generating new revenue streams.
Applying Product Development Thinking to Data
This quote from Zhamak Dehghani’s original article is key to understand the definition of data as a product:
“Domain data teams must apply product thinking […] to the datasets that they provide; considering their data assets as their products and the rest of the organization’s data scientists, ML and data engineers as their customers.”
Incorporating product development methodologies into data products can significantly boost their value and effectiveness. This approach involves several key strategies:
Market Analysis:
-
Understanding Internal Market Needs: Conduct a thorough analysis of the 'market' within the organization. Identify which departments or teams will use the data product and how it can add value to their operations.
-
Example: A financial department might need a data product for budget tracking and forecasting using data from finance-specific sources.
User-Centric Design:
-
Designing with the End-User in Mind: Data products should be tailored to the specific needs and challenges of their users.
-
Example: Creating a user-friendly dashboard for sales teams to track performance metrics.
Iterative Development:
-
Employing Agile Methodologies: Develop data products in iterative cycles, allowing for regular user feedback and necessary adaptations.
-
Continuous Improvement: This ensures that the data product evolves with changing user needs and remains relevant.
Quality Assurance and Testing:
-
Ensuring Data Accuracy and Usability: Conduct rigorous testing for data accuracy, usability, and performance.
-
Automated and Regular Checks: Implement automated checks for data quality, as data reliability is a cornerstone of a trustworthy data product.
Lifecycle Management:
-
Managing from Inception to Retirement: Data products, like traditional products, have a lifecycle. Effective management throughout this lifecycle is vital to maintain efficiency and relevance.
-
Adaptation and Scalability: Ensure that the data product is adaptable to changing business landscapes and scalable as per organizational growth.
Additional Considerations:
-
Discoverability and Accessibility: Make data products easily discoverable and accessible within the organization. A centralized data “store” or “marketplace”, such as a data catalog or Semantic Layer, can facilitate this.
-
Self-Describing and Interoperable: Include comprehensive metadata to make datasets self-describing and ensure they follow standard naming conventions for interoperability.
By treating data products as you would any other product, organizations can create data products that are not just functional but also foundational to driving informed decision-making and operational efficiency.
Data Products vs Data Assets
Understanding the distinction between data assets and data products is fundamental in the realm of data management. While these terms are often used interchangeably, they refer to distinct concepts:
-
Data Assets: These are the raw ingredients in the data ecosystem. Data assets can be any form of data collected or stored by an organization. This includes databases, spreadsheets, raw data file, etc. The key aspect of data assets is their potential; they are valuable resources that an organization holds but have not necessarily been refined or tailored for specific uses.
-
Data Products: In contrast, data products are the refined output created from data assets. They are the result of processing, organizing, and interpreting data assets to serve a specific purpose, be it for decision-making, operational use, etc. Data products are characterized by their readiness for use, having been cleaned, structured, and often enriched with context to make them immediately valuable and actionable.
The Transformation from a Data Asset to a Data Product
The journey from a data asset to a data product involves several critical steps:
-
Identification of Purpose: The transformation begins with identifying the purpose or the end goal for which the data is needed.
-
Data Processing and Refinement: Data assets undergo processing which may include cleansing, standardizing, aggregating, and enriching the data.
-
Contextualization and Customization: The data is then contextualized and customized according to the intended use, audience, and delivery platform.
-
Quality Assurance and Governance: Ensuring the data product adheres to quality standards, governance policies, and compliance requirements.
This transformation is not just a technical process but also a strategic one, where data is aligned with business objectives and user needs.
The Role of Data “Store” in Defining Data Products
Data stores (or “marketplaces”) play a critical role in the transformation of data assets into data products:
-
Centralized Access and Management: Much like a normal store or marketplace, data stores provide a centralized location for accessing data products. They facilitate the management, organization, distribution, and retrieval of data in a structured manner.
-
Transactional Nature: In an advanced data store, a data product will often acquire a transactional nature. This refers to the way data products are packaged, accessed, and utilized within the store. For instance, a data product might have unique identifiers (like SKUs in retail), metadata describing its content and use, subscription and delivery options, and terms of service.
-
Enabling Discoverability and Usability: By residing in a data store, data products become more discoverable and usable. Data stores enable users to browse, evaluate, and select data products that meet their specific requirements, much like shopping in a digital marketplace.
In summary, while data assets are valuable raw materials in an organization's data arsenal, data products are the refined, purpose-driven outputs that drive actionable insights and decisions. The transformation from a data asset to a data product is a nuanced process that involves not just technical refinement but also strategic alignment with business goals. Data stores augment this process by providing a structured environment that enhances the value, accessibility, and usability of data products.
Data Products vs Analytical Products vs AI Products vs Metadata Products
While data products, analytical products, AI products, and metadata products are closely related, they are not the same. The main differences lie in their purpose, composition, and use cases:
-
Data Products: These are the foundational elements consisting of data that has been processed, packaged, and curated for use. Data products provide the “building blocks” that can be used to generate insights or power data-driven applications.
-
Analytical Products: On the other hand, analytical products are the insights derived from data products. They represent the interpretation, analysis, or application of data to solve specific problems or answer questions. Examples include reports, dashboards, embedded analytics, etc.
- AI Products: These are sophisticated solutions that leverage artificial intelligence and machine learning algorithms to process data, learn patterns, and make predictions or decisions. AI products go beyond traditional analytical products by incorporating advanced techniques like natural language processing, computer vision, or deep learning. They often have the ability to adapt and improve over time based on new data and feedback. Examples include AI and machine learning models, chatbots, recommendation systems, autonomous vehicles, and predictive maintenance systems.
- Metadata Products: These are specialized products that provide information about other data and data-related assets within an organization. Metadata products help in organizing, understanding, and managing data more effectively. They typically include semantic layers, data catalogs, data lineage, data dictionaries, etc.
While data products, analytical products, AI products, and metadata products are all part of the data value chain, they serve distinct purposes and offer different levels of sophistication. Data products provide the foundation, analytical products offer insights, AI products deliver advanced, often autonomous solutions, and metadata products enhance the understanding, management, and utilization of data assets.
Each type of product builds upon the others in a complementary manner:
- Data products form the base layer, providing clean, structured, and reliable data.
- Analytical products use this data to generate human-interpretable insights and visualizations.
- AI products leverage both raw data and analytical insights to create more complex, predictive, and adaptive solutions.
- Metadata products span across all these layers, improving the discoverability, understanding, and governance of the entire data ecosystem.
By utilizing all four types of products, organizations can maximize the value they extract from their data assets, enabling more informed decision-making, improved operational efficiency, and the development of innovative data-driven products and services.
The 3 Layers of Data Product Development
Understanding the concept of a "data product" is essential, as it involves various layers and components that collectively turn a data asset into a data product.
Together, these three layers combine to form what we typically refer to as a “data product”, but it’s important to understand the distinct roles each layer plays in the process of data product development and delivery:
1. Foundational Layer: Master Data Products
Master Data Products serve as foundational pillars in data management, acting as a "single source of truth" for critical business entities, and ensuring consistency and reliability across various functions and applications within an organization. Master Data Products consist of dimension tables and fact tables, often referred to as "golden records”:
-
Fact Tables: Fact tables contain quantitative data about business operations. They store the metrics or measurements of business processes, such as sales transactions or inventory levels, often linked to dimension tables for context.
-
Dimension Tables: These tables contain descriptive attributes or dimensions of business entities. For instance, a customer dimension table might include customer IDs, names, addresses, and contact details.
Master Data Products are pivotal for ensuring consistency and accuracy across various business functions and analytical applications. They enable organizations to maintain standardized, reliable data that forms the backbone of their data environment.
2. Modeling Layer: Packaged Data Products
Packaged Data Products combine multiple Master Data Products in a structured way that optimizes data for complex queries and analytical tasks. These data products are built using various modeling concepts to create analytical structures:
Dimensional Modeling
Dimensional modeling is optimized for data warehousing and analytical querying, where data is structured into fact tables containing quantitative data and dimension tables containing descriptive attributes. This approach allows for fast, efficient querying and reporting by organizing data in a way that supports easy aggregation and slicing. Dimensional modeling is commonly used in business intelligence to create clear, understandable schemas for complex data analysis, such as star or snowflake schemas.
- Star Schemas: This structure consists of a central fact table that contains quantitative data (measures) and multiple dimension tables that provide context (descriptive attributes). The star schema is straightforward and optimized for fast querying and reporting.
- Snowflake Schemas: An extension of the star schema, the snowflake schema normalizes dimension tables into multiple related tables, which reduces redundancy but can complicate querying. This structure is used when there is a need for a more normalized data model to maintain data integrity.
OLAP (Online Analytical Processing) Cubes
An OLAP cube is built on top of the star schema (or snowflake schema) to enable multidimensional data analysis. The fact table in the schema provides the central quantitative data, while the dimension tables offer the descriptive context necessary for analysis. These tables are used to define the dimensions and measures within the OLAP cube, allowing for complex queries and data aggregation. The cube structure enables users to perform operations like slicing, dicing, drilling down, and rolling up, making it easier to explore and analyze data from various perspectives.
Tabular Models
Like star and snowflake schemas, tabular models organize data into tables and use in-memory columnar storage for high performance. They employ dimension and fact tables for logical data structuring but offer more flexibility and ease of management. Providing OLAP-like capabilities, tabular models enable fast aggregation, slicing, and dicing of data for advanced analytics. Commonly used in tools like Microsoft Power BI, they integrate well with traditional OLAP systems, combining structured data approaches with modern in-memory processing for efficient business intelligence.
Each of these approaches helps to structure data in ways that optimize it for analytical tasks, enabling efficient querying, aggregation, and exploration of large datasets. The choice between these methods often depends on factors such as the specific analytical needs, the volume of data, the desired query performance, and the tools being used for analysis.
Packaged Data Products built using these methods create a foundation for advanced analytics, business intelligence reporting, and data-driven decision-making processes within an organization.
Business Metrics
Analytical structures like star schemas, snowflake schemas, OLAP cubes, and tabular models organize data into clear, logical formats, making it easy to identify and extract data needed for business metrics. They play a pivotal role in business metrics creation by aggregating KPIs (facts) such as "total sales" or "number of purchases" across various dimensions like time, geography, or product categories. These structures empower organizations to create consistent, organization-wide metrics, derive meaningful insights, and make informed decisions based on the aggregated data.
3. Semantic Layer: Curated Data Products
The Semantic Layer takes the Packaged Data Products (such as OLAP cubes and tabular models) from the Modeling Layer and further refines them to create business-friendly "Curated Data Products".
These Curated Data Products are created by carefully selecting a subset of Packaged Data Products from the Modeling Layer and delivering them to business users, analysts, and other stakeholders in a format that aligns with familiar business concepts and terminology.
Once created, these business-friendly Curated Data Products (sometimes called "semantic models") can be deployed to various visualization tools and reports, making the data easily accessible and usable for users of all skill levels. In many business intelligence contexts, when we discuss "data products", we are often referring to these curated, business-ready outputs.
For example, the data team at a retail company might create a Curated Data Product to give its sales team a model of their sales data that's easy to navigate and understand. This curated dataset compiles key sales metrics from various sources, such as online sales, in-store transactions, and customer demographics.
The sales team can then use this Curated Data Product to gain real-time insights into sales performance, customer behavior, regional sales trends, etc. By deploying this data product to easy-to-understand dashboards and reports, they can make informed decisions, optimize product offerings, target specific customer segments, and ultimately boost sales revenue.
Curated Data Products are instrumental in democratizing data access within organizations while ensuring governance and data quality. They enable end-users of all technical levels to perform analyses and derive insights. This democratization of data not only streamlines workflows but also fosters a data-driven culture within the organization.
Together, these three layers combine to form the comprehensive concept of a "data product." It's important to recognize the distinct roles each layer plays in the development and delivery of data products, creating a robust ecosystem that empowers organizations to harness the full potential of their data.
The Semantic Layer as a Centralized “Store” for Data Products
The semantic layer acts as a centralized “store” for a company's data products, making them easily accessible to business users, analysts, and other stakeholders. The semantic layer provides this unified view of data products by abstracting the complexity of underlying data structures, standardizing metrics and definitions, and presenting them in business-friendly terms, ensuring consistency and accuracy across various reports and dashboards.
By organizing and storing data products within the semantic layer, business users, analysts, and other stakeholders can easily access and utilize them. This approach enhances data usability and fosters a more intuitive interaction with the data, enabling more informed decision-making and efficient data analysis.
Why Do You Need Data Products?
Data products are not just a technical concept in the realm of data management; they are a strategic asset that brings multifaceted benefits to an organization. Understanding why data products are essential can be categorized into three primary areas:
Business Advantages:
-
Improved Decision Making: Data products provide structured, reliable, and actionable insights. This clarity aids in making informed decisions, enhancing business strategies, and driving growth.
-
Enhanced Customer Experiences: By leveraging data products, companies can gain a deeper understanding of customer behavior, preferences, and needs. This leads to more personalized and effective customer engagement.
-
Increased Operational Efficiency: Data products streamline operational processes by providing accurate and timely data. This efficiency reduces costs and improves productivity.
-
Innovative Product and Service Development: The insights derived from data products can lead to the creation of new and improved products and services, keeping the business competitive and relevant in the market.
Data Management Advantages:
-
Standardization and Consistency: Data products ensure a standardized approach to handling data, maintaining consistency across various business units.
-
Quality and Reliability: With structured processing and governance, data products are reliable and of high quality, reducing the risk of errors and misinformation.
-
Simplified Access and Usability: Data products are designed to be accessible and user-friendly, enabling a wider range of users to leverage data for their specific needs without requiring extensive technical expertise.
-
Enhanced Data Governance: They facilitate better data governance practices by defining clear standards and policies for data usage, security, and compliance.
Organizational Advantages:
-
Fostering a Data-Driven Culture: Data products democratize data access within an organization, encouraging a culture where decisions are based on data-driven insights.
-
Cross-Functional Collaboration: They enable various departments to collaborate more effectively, as data is presented in a format that is easily understandable and actionable for different teams.
-
Scalability and Flexibility: Data products allow organizations to scale their data initiatives efficiently. They can be adapted and expanded as the business grows and its needs change.
-
Risk Mitigation: By providing accurate and up-to-date information, data products help in identifying potential risks and challenges early, allowing for timely intervention.
Building a Data Product: A Step-by-Step Guide
Creating a data product involves a series of steps, each critical to ensuring that the final product is effective, reliable, and valuable. This guide outlines the key steps and best practices in developing data products, including the integration of tools and technologies like TimeXtender:
Step 1. Understand Internal Needs
Identify Internal “Customer” Requirements: Begin by conducting interviews with internal teams to gain a deep understanding of their data needs. Engage in open and collaborative discussions to uncover pain points and data gaps that need to be addressed.
Define Objectives: Collaborate closely with stakeholders to set clear, achievable, and measurable objectives. These objectives should be mapped to specific business processes and desired outcomes, ensuring alignment with organizational goals.
Step 2: Data Collection and Preparation
Gather Data: Identify and access relevant data sources, which may include internal databases, CRM systems, and external third-party data. Utilize data integration tools to streamline the collection process and ensure data accessibility.
Cleanse, Standardize, and Transform Data: Apply data cleansing techniques to eliminate duplicates, correct errors, and enhance data quality. Standardize data formats and categorizations to ensure consistency across datasets, improving overall data reliability.
Step 3: Utilize TimeXtender for Data Integration
Holistic Data Integration: Leverage TimeXtender to automate the integration of data from diverse sources, eliminating manual data handling errors and ensuring a streamlined process. TimeXtender's metadata-driven approach accelerates the entire data lifecycle, from data ingestion and transformation to modeling and delivery.
Step 4: Develop and Model the Data Product
Data Modeling: Create dimensional data models using TimeXtender’s intuitive, drag-and-drop interface, aligning them with internal reporting and analysis requirements. Design data structures that efficiently serve the needs of stakeholders.
Iterative Development: Develop initial prototypes and engage in iterative feedback loops with end-users. Continuously test and refine the data model based on user input, ensuring that it evolves to meet evolving needs and expectations.
Step 5: Create User-Friendly Interfaces
Develop Interfaces and Dashboards: Design intuitive and user-friendly dashboards that present data in a visually accessible format. Incorporate visualization tools to enhance data interpretation and provide users with actionable insights.
Step 6: Implement Governance and Compliance
Ensure Data Governance: Establish data governance committees or teams responsible for overseeing data usage and security. Implement policies and procedures for data access, quality control, and regulatory compliance to ensure data integrity and security.
Step 7: Beta Testing and Feedback
Internal Testing: Engage a diverse group of users for beta testing to gather comprehensive feedback. Monitor usage patterns and collect qualitative feedback to identify areas for improvement. Beta testing helps uncover issues and ensures that the data product aligns with user expectations.
Step 8: Launch and Train
Rollout: Plan a phased rollout strategy, beginning with a pilot group to test the data product in a controlled environment. Monitor system performance and user engagement post-deployment to address any unforeseen issues promptly.
Training and Support: Provide comprehensive training sessions and documentation to enable users to make the most of the data product. Establish a support system for ongoing assistance and troubleshooting, ensuring users have the resources they need for a seamless experience.
By following these steps and leveraging the power of tools like TimeXtender, organizations can create effective, efficient, and valuable data products that cater to the specific needs of their internal users, driving informed decision-making and operational excellence.
The Role of Data Fabric in Data Product Development
What is Data Fabric?
Data fabric is a holistic, flexible, and scalable architecture designed to maximize the value of data within an organization. It is not a singular tool, but rather an innovative framework for integrating various tools, systems, and processes to create a seamless and unified data environment.
The core idea behind data fabric is to provide a holistic view of all data across the organization, regardless of its location or format. This approach enables seamless data ingestion, access, preparation, sharing, and analysis, facilitating more efficient and effective data management.
Key Components of a Data Fabric:
-
Unified Metadata Framework: Acts as the central nervous system, coordinating and managing data across various sources and systems. It ensures consistency and accessibility of data, enabling easier integration and analysis.
-
Data Sources: Represents the diverse origins of data, including internal databases, cloud sources, IoT devices, and third-party data. The fabric integrates these varied sources for a holistic data view.
-
Data Ingestion: Involves the process of importing, transferring, loading, and processing data from these sources.
-
Data Preparation: Entails cleansing, transforming, and enriching data to make it suitable for analysis. This step is vital for ensuring data accuracy and usability.
-
Data Delivery: Focuses on providing data to end-users in an accessible format, often through APIs, data services, or visualization tools, enabling effective decision-making.
-
Data Quality: Ensures the accuracy, consistency, and reliability of the data throughout its lifecycle. This is critical for maintaining the integrity of data products.
-
Data Observability: Involves monitoring the health and performance of the data ecosystem, along with documentation and data lineage, ensuring data reliability and operational efficiency.
-
Data Storage: Refers to the methods and architectures used to store data securely and efficiently, whether in on-premises databases, cloud storage, or hybrid systems.
-
AI-Powered DataOps: Leverages artificial intelligence to automate and optimize data operations, enhancing the speed and efficiency of data processing and analytics.
-
Security and Governance: Encompasses the practices and technologies used to protect data from unauthorized access and ensure compliance with regulations and internal policies.
Each component of the data fabric is essential for ensuring the data product development process is streamlined, efficient, and yields high-quality, reliable data products.
Data Mesh: A Decentralized Approach to Data Product Management
What is Data Mesh?
Data Mesh is an innovative organizational approach to managing and utilizing data. It is important to understand that Data Mesh is neither a specific technology nor an architectural framework; rather, it is a set of organizational principles rooted in "domain-driven design".
Key Characteristics of Data Mesh:
-
Organizational Principles, Not Technology: Data Mesh emphasizes a philosophical shift in how data is perceived and handled within an organization. It moves away from viewing data as a mere byproduct of applications or systems and instead treats it as a valuable asset that requires dedicated attention and stewardship.
-
Treating Data as a Product: Data Mesh encourages each domain to develop and manage its data as a "product" with clear value propositions. This means ensuring that the data is easily accessible, reliable, and presented in a way that is understandable and usable by other domains or teams within the organization.
-
Decentralization of Data Management: In a Data Mesh approach, the responsibility for data product development is decentralized and distributed among various domain teams. Each team takes ownership of their own data, treating it as a product that needs to be well-maintained, documented, and made usable for others within the organization.
-
Cross-Functional Data Teams: Data Mesh encourages the formation of cross-functional teams within each domain. These teams comprise data engineers, data scientists, and business experts who work together to ensure that their data products are relevant, reliable, and accessible.
Data Mesh, Data Fabric, and Data Products
While these terms are interconnected, they are very different concepts, so it’s important to be clear on the definitions and distinctions of each:
Data Mesh and Data Fabric:
-
Complementary Concepts: Data Mesh and Data Fabric are complementary, with Data Mesh focusing on the organizational design, while Data Fabric provides the technological framework to implement it.
-
Data Fabric as an Enabler: Data Fabric acts as the technological enabler for Data Mesh, providing the centralized infrastructure and governance needed for efficient data integration and management across decentralized domains.
Data Fabric and Data Products:
-
Integration and Management Framework: Data Fabric provides a comprehensive framework for integrating and managing data across various sources and systems. This framework is essential for creating Data Products, which are specific sets of data organized and optimized for easy consumption and analysis.
-
Enabling High-Quality Data Products: The integration capabilities of Data Fabric ensure that Data Products are of high quality. They are reliable, consistent, and ready for use in various business applications. This reliability is crucial for making informed decisions based on data.
-
Facilitating Access and Use: Data Fabric not only organizes data but also makes it more accessible. This accessibility is key for different departments and teams within an organization to use Data Products effectively. It allows for a more widespread use of data, enhancing data-driven culture in the organization.
-
Supporting Customization and Relevance: With Data Fabric, Data Products can be tailored to meet the specific needs of different users and scenarios. This customization ensures that the data is not only accessible but also relevant and valuable for its intended purpose.
Data Mesh and Data Products:
-
Empowering Domains: Data mesh empowers domains to develop data products that are tailored to their specific needs, while also being valuable for other domains and stakeholders.
-
Product-Centric Approach: Data Mesh’s product-centric approach to data means that each domain's data is designed, managed, and utilized with specific users and use cases in mind.
-
Inter-Domain Collaboration: This approach fosters collaboration and data sharing across domains, enhancing the overall data literacy and data-driven decision-making capabilities of the organization.
By combining the principles of Data Mesh with the technological capabilities of Data Fabric, and focusing on the development of domain-specific Data Products, organizations can achieve a more agile, user-focused, and collaborative data environment.
TimeXtender: A Holistic Solution for Building Data Fabric, Data Products, and Data Mesh
TimeXtender offers a unique, dual-faceted solution that integrates two essential components for modern data management: Data Fabric Builder and Data Product Builder:
This integration provides a holistic solution that caters to the varied needs within data teams, ensuring security, efficiency, and alignment throughout data integration and analytics workflows.
1. Data Fabric Builder
The Data Fabric Builder is designed for Data Movers, such as Data Architects, Data Engineers, and Database Administrators, who are responsible for building and maintaining an organization’s data infrastructure.
This Data Fabric Builder focuses on building a robust, secure data infrastructure that serves as the foundation for analytics and AI. It utilizes metadata to unify the entire data stack, crafting a comprehensive and interconnected data fabric. This approach dramatically accelerates the process of building data infrastructure up to 10 times faster than traditional methods.
Key Features:
-
Rapid Infrastructure Development: Develop your data fabric up to 10 times faster, making it easier to respond to changing business requirements.
-
Robust and Secure: Ensures a secure and reliable foundation for analytics and AI, essential for the IT teams who prioritize stability and security in data management.
-
Future-Proof: With the ability to easily adapt and expand, the Data Fabric Builder ensures you don’t get held back by outdated technology or vendor lock-in.
2. Data Product Builder
On the other side, we have the Data Product Builder, tailored for Data Users like business intelligence (BI) experts and analysts.
The Data Product Builder is all about agility in creating and delivering actionable, business-ready data as fast as possible. It’s about empowering users with an intuitive, low-code tool that democratizes data preparation, access, and analytics. This democratization allows users to easily transform and model data, swiftly access datasets, and generate reports and dashboards without relying heavily on IT support.
Key Features:
-
Low-Code User Interface: A simple, intuitive interface allows users to create data products without needing deep technical expertise.
-
Speed and Agility: Deliver data products up to 10 times faster, enabling rapid decision-making and business agility.
-
Empowering Data Users: Puts the power of data in the hands of the users who need it most, supporting a decentralized approach to data management.
A Single, Holistic Solution for Data Integration
This dual approach addresses the distinct needs of Data Movers and Data Users, ensuring that both can perform their roles efficiently through two specialized capabilities that are seamlessly integrated into a single, holistic solution.
Supporting a Decentralized Data Mesh Approach
TimeXtender serves as a holistic solution that addresses both the foundational aspects of data infrastructure and the tooling needs of data product creation. Its dual-purpose functionality ensures that your organization can seamlessly tackle infrastructure and governance concerns while also meeting the decentralized tooling needs essential for implementing a robust data mesh strategy:
-
Data Fabric Builder for Centralized Infrastructure and Governance: TimeXtender's Data Fabric Builder is the backbone of your data infrastructure and governance strategy. It provides a centralized platform that seamlessly integrates data from diverse sources, ensuring a unified view of your organizational data. With robust data governance capabilities, it allows you to implement data policies, track data lineage, and enforce security protocols, safeguarding data integrity and compliance with industry regulations.
-
Data Product Builder for Decentralized Data Product Development: Simultaneously, TimeXtender’s Data Product Builder is uniquely equipped to cater to the decentralized tooling requirements inherent in a data mesh approach. It offers an intuitive, drag-and-drop interface that enables business intelligence experts, analysts, and domain experts to collaboratively build and maintain data products without the need for extensive coding or specialized technical skills. This democratization of data product creation fosters agility within your organization, as teams can independently develop and deploy data products, reducing bottlenecks and accelerating time-to-insights.
In summary, TimeXtender's holistic capabilities bridge the gap between infrastructure and governance concerns, and the decentralized tooling needs of a successful data mesh strategy.
Try Out Our Data Product Builder for Free!
Click here to get started with a FREE trial and try out all the capabilities you need to create powerful data products and unlock the full potential of your data, without a large team or a complex stack of expensive tools!