4 min read
Why Data Transformation Is Vital for Effective Data Integration
Written by: Aaron Powers, Content Marketing Specialist - November 22, 2023
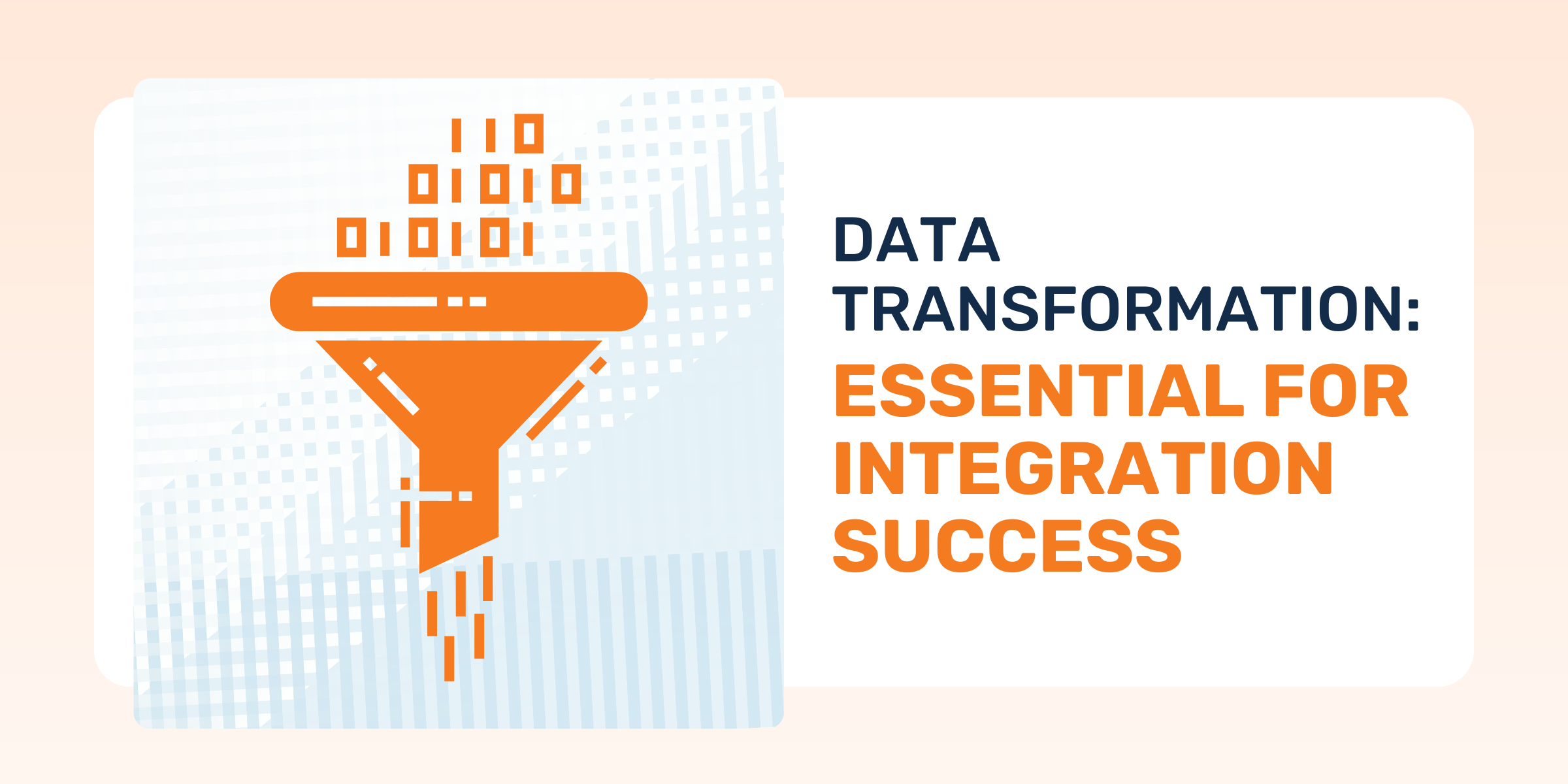
In this modern era of data abundance (and often overload), seamless integration of information is vital for businesses hoping to gain a competitive edge. Data integration, the process of combining and unifying various data sources, forms the bedrock of informed decision-making. However, data transformation is a crucial aspect that’s often overlooked. In this post, we'll uncover not only why data transformation is important, but why it’s crucial for effective data integration.
Understanding Data Transformation
Definition of Data Transformation
Data transformation involves converting and manipulating raw data into a format that is compatible and useful for the target system. It's the key to unlocking the true potential of data in any integration process. Check out our previous post on data transformation to learn more!
Role of Data Transformation in the Data Integration Process
Without data transformation, integrating disparate data sources would be like trying to fit a square peg into a round hole. Incompatible formats and structures lead to a jumbled, incomprehensible dataset, that can't be understood or analyzed properly.
Data Transformation Enhances Data Quality and Usability
By cleaning, enriching, and standardizing data, transformation plays a pivotal role in enhancing data quality. It removes inconsistencies, corrects errors, and fills in missing information, resulting in a dataset that’s more accurate and reliable, which is necessary for informed decision-making.
Potential Pitfalls of Using Raw Data in Integration Processes
Consider a scenario where a retail company is trying to merge customer data from different departments or different store locations. Without proper transformation, names may be formatted differently, addresses could be incomplete, and contact information might be missing. These inconsistencies can lead to inaccurate analyses and flawed business decisions, which is something no company wants.
Benefits of Data Transformation in Data Integration
Improved Data Quality and Consistency
Data transformation acts as a filter, sifting out inconsistencies and discrepancies. This results in a dataset that is not only accurate, but also consistent, forming a reliable foundation for subsequent analyses.
Enhanced Compatibility Between Different Data Sources
Different systems often speak different "languages" when it comes to data. Data transformation bridges this linguistic gap, enabling seamless communication between disparate sources. It’s your universal translator, ensuring there’s no misunderstanding between different data sources.
Streamlined Data Processing and Analytics
Think of transformed data like a well-organized library. Every book, journal, and reference material is labeled with a standardized system and placed on its proper shelf. This allows for anyone to quickly retrieve and analyze the data, saving valuable time and resources. Data transformation arranges and structures information in a manner that creates streamlined data processing and analytics. It takes raw, potentially disorganized data and refines it into a cohesive, standardized format. Users can navigate the transformed dataset with ease, swiftly extracting the insights they need.
Common Data Transformation Techniques
Popular Data Transformation Methods
Extract, Transform, Load (ETL) and data wrangling are two of the most prevalent and popular data transformation techniques. ETL focuses on moving and transforming data in batches, while data wrangling involves the process of cleaning and preparing data for analysis.
- ETL:
- Operates on data in batches
- Primarily used for structured data
- Involves predefined, structured pipelines
- Typically used in traditional data warehouses
- Emphasizes data movement between systems
- Data Wrangling:
- Operates on data in a more flexible, interactive manner
- Suitable for both structured and semi-structured data
- Involves exploratory data manipulation
- Often used in data preparation for analysis or machine learning
- Focuses on data cleaning, enrichment, and transformation in a more ad-hoc fashion.
When deciding between ETL and data wrangling for data transformation, the choice often hinges on the specific requirements of the task at hand and the nature of the data involved. ETL is the better choice when dealing with large volumes of structured data that require a systematic and batch-oriented approach. Its predefined, structured pipelines make it efficient for moving and transforming data between systems, making it well-suited for traditional data warehouses. This is what TimeXtender offers with our holistic data integration tool, regardless of your data storage solution. As for data wrangling, it shines in scenarios where flexibility and interactivity are paramount. It excels in handling both structured and semi-structured data, allowing for more exploratory and ad-hoc manipulation.
Best Practices for Effective Data Transformation
Data Profiling and Understanding Source Data
Before beginning any data transformation journey, it's important to understand the source data thoroughly. Profiling the data helps identify potential challenges and informs the choice of transformation techniques. Data profiling involves carefully examining the characteristics, structure, and potential quirks of the raw data. This process unveils crucial insights, such as data types, distributions, and anomalies.
Choosing the Right Transformation Techniques for Specific Use Cases
Not all transformation techniques are created equal. Tailoring the approach to the specific use case ensures the transformed data aligns with the intended business objectives. This precision in technique selection ensures the transformed data aligns not only with the immediate needs of the project, but also with the broader strategic goals of the organization.
Testing and Validation of Transformed Data
Just as an airline pilot conducts pre-flight checks, data transformation should undergo rigorous testing and validation. This step ensures the transformed data meets the desired quality and accuracy standards. Thorough testing helps identify and fix any discrepancies or anomalies that may have emerged during the transformation process. This precise validation process provides confidence the transformed data is accurate and ready to empower informed decision-making and drive positive business outcomes.
TimeXtender's Approach to Data Transformation
SimplifY Efficient Data Transformation
At TimeXtender, we understand the necessary role of data transformation in the integration process. Our data integration tool streamlines and automates this crucial step, empowering users to transform data with speed and precision.
Successful Transformations with TimeXtender
TimeXtender has contributed to plenty of real-world success stories that serve as a testament to the effectiveness of our approach to data transformation. From accelerating time-to-insight to improving data quality, our data transformation and integration tool has made a tangible impact on businesses worldwide:
- Blue Lagoon Journey with TimeXtender
- This Icelandic wonder of the world democratized data access and moved away from data silos that were primarily for financial purposes and rarely updated.
- PEI Media Chooses TimeXtender for Digital Transformation
- PEI needed a data solution that helped them overcome disparate data sources and react to changes in the business quickly and accurately.
- Pathé Implements TimeXtender
- The largest cinema chain in the Netherlands needed an innovative way to create a data warehouse following the impact of COVID-19.
The Unsung Hero
In the world of data integration, data transformation is the unsung hero that creates and ensures the seamless flow of information. It has a significant impact on data quality, compatibility, and usability. As you begin your next data integration journey, remember – effective transformation is the key to unlocking the true potential of your data.
Ready to revolutionize your data integration processes? Explore TimeXtender's holistic data integration solution and experience the power of seamless, efficient data transformation. Request your free trial and unlock the full potential of your data now!