8 min read
Data Movers vs Data Users: Bridging the Divide Within the Data Team
Written by: Diksha Upadhyay - April 20, 2023
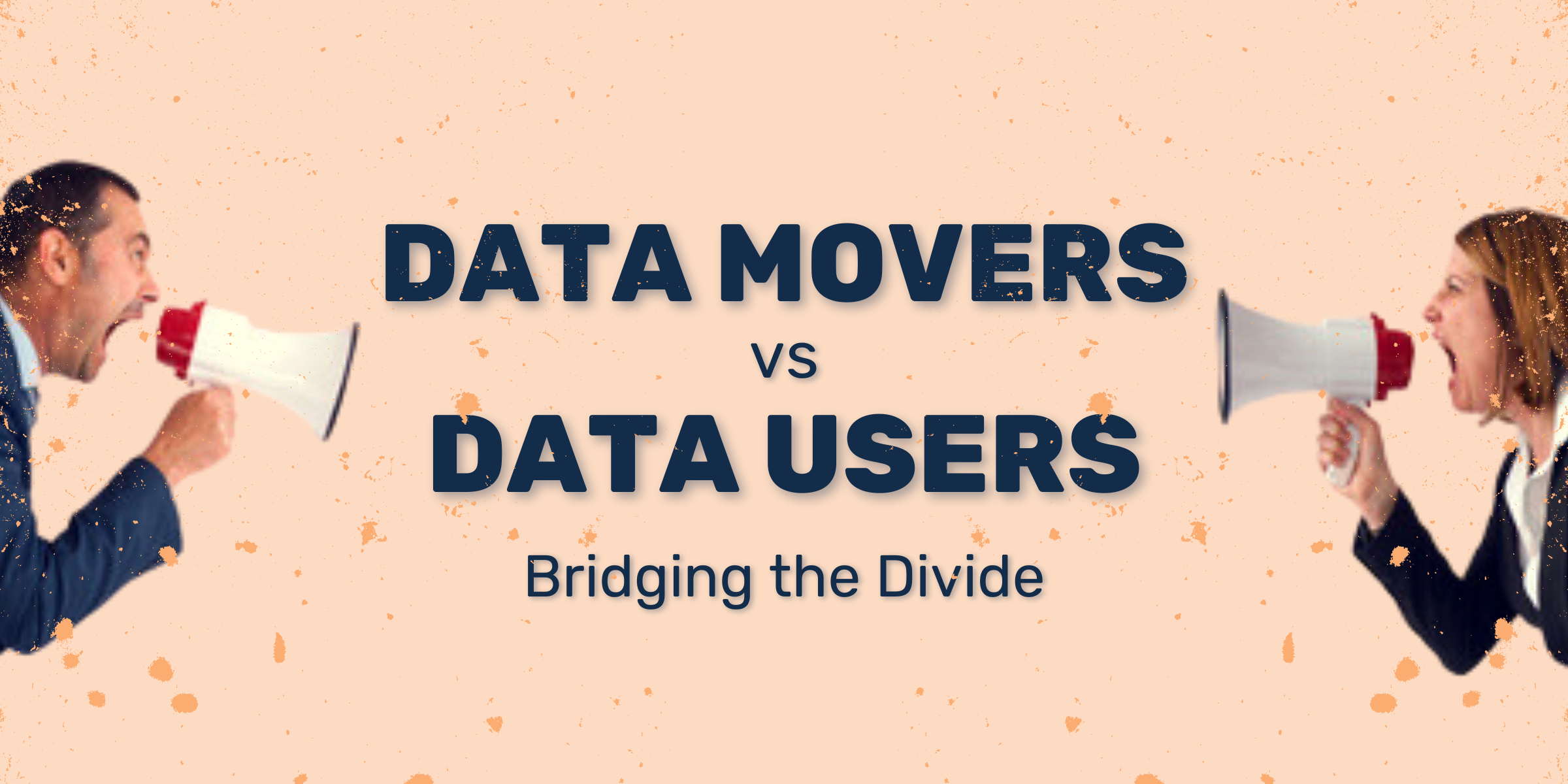
Scroll to the bottom for a summary.
In just the past decade, data teams have gone from being the nerdy backroom dwellers to the cool kids at the forefront of business. The transformation of these teams has been swift and dramatic. They’re not only handling more data but also facing new challenges, like privacy concerns and the ethical use of AI.
At the same time, the tools and platforms for handling data have been evolving at breakneck speed. From next-gen machine learning frameworks to advanced data visualization, these modern marvels have made it easier for data teams to dive deep into the ocean of information and extract meaningful insights.
However, not everything is sunshine and rainbows in the world of data. As teams have expanded and the stakes have risen, a growing number of problems have also begun to emerge.
The Changing Landscape of Data Team Structures
Ten years ago, data teams faced a very different set of challenges than they do today. Back then, the primary challenge for data teams was storage. Data storage was extremely expensive, and businesses had to carefully choose what data they would store, as well as how long they would keep it.
In addition to the storage limitations, data teams faced the challenge of managing data using outdated and clunky tools. Data analysis and processing required specialized technical skills, and the tools available at the time were not user-friendly. This often led to a bottleneck in data processing, as data teams struggled to keep up with the demands of the business.
The emergence of cloud computing and advancements in technology have transformed the data landscape in recent years. Today, data teams have access to massive amounts of storage at a fraction of the cost. They also have access to modern tools and platforms that make data analysis and processing faster and more efficient.
The past few years for data teams have been exhilarating, challenging, and downright transformative. The data landscape has evolved dramatically, giving rise to two distinct groups within the data team:
1. Data Movers
Data Movers are the data architects, engineers, developers, database administrators, etc.
These are the overworked, underpaid, unsung heroes of the data world, busting their butts to collect, move, and manage the avalanche of information that keeps piling up, all while ensuring governance, security, and compliance with industry rules and regulations.
Data Movers mostly view data as a byproduct of business processes that they must wrangle, store, and provide secure access to, but not necessarily something they’re tasked with turning into business value (but mostly because they simply don’t have the time).
Unfortunately, Data Movers are drowning in a sea of requests, feeling undervalued and burned out. In fact, new research has found that 97% of data engineers are feeling burned out in their day-to-day jobs, and 79% are considering leaving the industry altogether.
2. Data Users
Data Users are the data scientists, data analysts, analytics engineers, BI architects, BI developers, etc.
These are the dreamers, the idea generators, and the ones who see data as the raw material that can be molded into something valuable for the business.
Data Users are much closer aligned to the needs of the business and are constantly on the lookout for ways to clean, refine, and organize data to create a treasure trove of insights that can propel the business forward.
Unfortunately, Data Users are often frustrated by the seemingly insurmountable barrier between them and the pristine, perfectly organized data sets of their dreams.
The Growing “Data Divide” and Its Impact on the Business
As these roles continue to specialize, a “Data Divide” is growing between these two groups. As you can see, these two groups view data fundamentally differently:
- Data Movers are focused on the logistics of managing and securing data.
- Data Users are focused on extracting insights from data to drive business value.
This divide is more than just a cultural or philosophical difference — it’s causing real problems for businesses trying to harness the power of their data:
- Data swamps: Data swamps are created when data is collected and stored without a clear strategy or plan for how it will be organized, modeled, and used. This often occurs when Data Movers are overwhelmed with requests and don’t have the time to do proper data modeling, resulting in a jumbled mess that Data Users struggle to make sense of. Data swamps are a problem because they make it nearly impossible for Data Users to find the data they need, let alone extract meaningful insights from it.
- Communication barriers: Communication barriers are another consequence of building a data swamp. Data Movers and Data Users end up having different definitions for their data and lack a shared language (semantic model) for understanding their data. This lack of shared understanding often leads to misinterpretation of data, mistakes in analysis, and conflicting recommendations, which can ultimately harm the business.
- Inaccessible insights: Inaccessible insights are another problem that arises from the growing data divide. With data teams struggling to communicate effectively and data swamps making it difficult to find and extract valuable information, businesses are missing out on key insights that could drive growth and innovation. In some cases, valuable insights may be buried in data that Data Movers have collected but haven’t had the time to properly model, while in others, Data Users may not even be aware of the existence of relevant data because it’s buried in a data swamp.
- Slower decision-making: The lack of communication and difficulty in accessing data insights can slow down the decision-making process. When the data is not effectively translated into insights, it becomes challenging for businesses to make quick decisions that can propel them forward.
- Missed opportunities: When the Data Movers and Data Users are not aligned, businesses can miss out on valuable opportunities for growth, cost-savings, and increased efficiency. With valuable data insights hidden, businesses may not be able to recognize new revenue streams, cost-saving measures, or potential areas of improvement.
- Stunted growth: Ultimately, the growing divide between Data Movers and Data Users can lead to stunted growth for businesses. When data insights are not accessible, and communication breakdowns persist, businesses cannot innovate or optimize their operations, leading to missed opportunities and ultimately stagnation.
As Data Movers and Data Users struggle to find common ground, the entire data ecosystem suffers. If the data team itself can’t even get on the same page when it comes to defining and using data, what hope does the rest of the business have?
It’s time to bridge the divide and start working together to extract those meaningful insights and drive the business forward.
A New Hope: The Future of Data Movers and Data Users
So, what’s next for the Data Movers and Data Users? Are they going to keep going their separate ways, or is there hope for reconciliation?
Well, the answer isn’t as clear-cut as you might think.
Return of the Data Analyst
One thing that’s clear is that the traditional data analyst role is making a comeback. As businesses struggle to bridge the divide within the data team, the need for a middle ground is becoming increasingly important.
Enter the data analyst.
Data analysts have been around for years, but in recent years, they’ve taken a back seat to the more specialized roles within the data team. However, with the growing data divide, the need for a more well-rounded data professional is becoming apparent.
Data analysts are uniquely positioned to bridge the gap between Data Movers and Data Users. They have a strong understanding of data modeling and analysis, but they also have the communication skills necessary to translate data insights into actionable business recommendations.
By combining technical skills with business acumen, data analysts can help Data Movers better understand the needs of the business, while also helping Data Users navigate the complexities of data modeling and analysis.
The Rise of Analytics Engineering
With the growing amount of data being collected and stored, Data Movers simply don’t have the time to properly transform and model data, which results in data swamps that are difficult for Data Users to navigate.
As a result, Data Users are starting to take matters into their own hands. They are becoming more technical and using modern tools like dbt and TimeXtender to do their own data transformation and modeling. This allows them to bypass the bottleneck of Data Movers and create the data sets they need to extract the insights they desire.
In doing so, Data Users are demanding more respect and recognition for their specialized skills. They are using a new title, “analytics engineer”, that gives them comparable prestige to that of their colleagues in data engineering.
Analytics engineers are data professionals who bridge the gap between both sides of the data team. They work closely with both Data Movers and Data Users to understand the needs of the business and ensure that data is properly collected, stored, and analyzed. They have a deep understanding of business needs, while also being technical experts who can both design and build data pipelines that are optimized for analysis.
The Last Data Engineer
While the rise of analytics engineers is an exciting development, it doesn’t mean that the role of data engineer is going away anytime soon. In fact, the demand for skilled data engineers is only going to increase as the amount of data being collected continues to grow.
Data engineers will still play a critical role in building and maintaining the infrastructure required to collect, store, and process massive amounts of data. However, they will also need to become more business-savvy and work more closely with Data Users to ensure that the data being collected is relevant and useful for the business.
As the data landscape continues to evolve, the role of the data engineer will become more specialized. They will need to become experts in specific areas like data security, governance, and compliance. They will also need to work closely with other data professionals like analytics engineers and data scientists to ensure that the data being collected is properly modeled and analyzed.
Uniting the Data Team with a Holistic Solution for Data Integration
Many data analysts and analytics engineers have high hopes for various tools in the “modern data stack”, such as dbt. They believe that these tools can solve all of their data transformation and modeling problems and bridge the Data Divide between Data Movers and Data Users.
However, the reality is that these tools can often create more fragmentation and complexity, exacerbating the Data Divide even further.
The Modern Data Stack is Broken
We’ve written at length here about the obstacles that have caused the modern data stack to fail at delivering on its most basic promises.
“The Modern Data Stack ended up solving mostly engineering challenges related to cost and performance, generating more problems when it comes to how the data is used to solve business problems.
The primary objective of leveraging data was and will be to enrich the business experience and returns, so that’s where our focus should be.”
– Diogo Silva Santos, Senior Data Leader
To overcome these challenges, data teams need to take a holistic approach to data integration that focuses on aligning around business objectives and implementing a unified metadata framework to create a semantic layer that maximizes data access, observability, and usability.
Meet TimeXtender, the Holistic Solution for Data Integration
The so-called “modern” data stack traces its origins back to outdated architectures designed for legacy systems. We believe it’s time to reimagine what’s possible through simplicity and automation.
Meet TimeXtender, the holistic solution for data integration.
TimeXtender provides all the features you need to build a future-proof data infrastructure capable of ingesting, transforming, modeling, and delivering clean, reliable data in the fastest, most efficient way possible — all within a single, low-code user interface.
You can’t optimize for everything all at once. That’s why we take a holistic approach to data integration that optimizes for agility, not fragmentation. By using metadata to unify each layer of the data stack and automate manual processes, TimeXtender empowers you to build data solutions 10x faster, while reducing your costs by 70%-80%.
TimeXtender is not the right tool for those who want to spend their time writing code and maintaining a complex stack of disconnected tools and systems.
However, TimeXtender is the right tool for those who simply want to get shit done.
From disconnected tools to Holistic Solution
Say goodbye to disconnected tools and systems. Say hello to a holistic solution for data integration that’s optimized for agility.
Data teams at top-performing organizations such as Komatsu, Colliers, and the Puerto Rican Government are already taking this new approach to data integration using TimeXtender.
Start Building Data Solutions 10x Faster with TimeXtender
Start your Free Plan today to unify your data stack, automate data integration processes, and build data solutions 10x faster.
Summary:
- The data landscape has evolved dramatically in recent years, giving rise to specialized roles within data teams.
- There are two distinct groups within the data team: Data Movers (data architects, engineers, developers, database administrators, etc.) and Data Users (data scientists, data analysts, analytics engineers, BI architects, BI developers, etc.).
- The growing “Data Divide” between Data Movers and Data Users is causing real problems for businesses trying to harness the power of their data.
- The problems caused by the Data Divide include data swamps, communication barriers, inaccessible insights, slower decision-making, missed opportunities, and stunted growth.
- Data analysts are making a comeback as businesses look for a “middle man” to facilitate communication between Data Movers and Data Users.
- Analytics engineers are becoming more specialized. These are data analysts with technical skills who can bridge the gap between both sides of the data team.
- The role of data engineer is still critical in building and maintaining the infrastructure required to collect, store, and process massive amounts of data. However, their role is going to evolve a lot over the coming years.
- To overcome the challenges of the modern data stack, data teams need to take a holistic approach to data integration that focuses on aligning around business objectives, along with implementing a unified metadata framework to create a semantic layer that maximizes data access, observability, and usability.
- TimeXtender is a holistic solution for data integration that provides all the features needed to build a future-proof data infrastructure capable of ingesting, transforming, modeling, and delivering clean, reliable data in the fastest, most efficient way possible — all within a single, low-code user interface.
- TimeXtender empowers data teams to build data solutions 10x faster, while reducing costs by 70%-80%.
Haven’t shared this post yet? Be a lot cooler if you did…