6 min read
Data Divide Part 2: How AI Will Accelerate the Data Divide
Written by: Micah Horner, Product Marketing Manager, TimeXtender - January 4, 2024
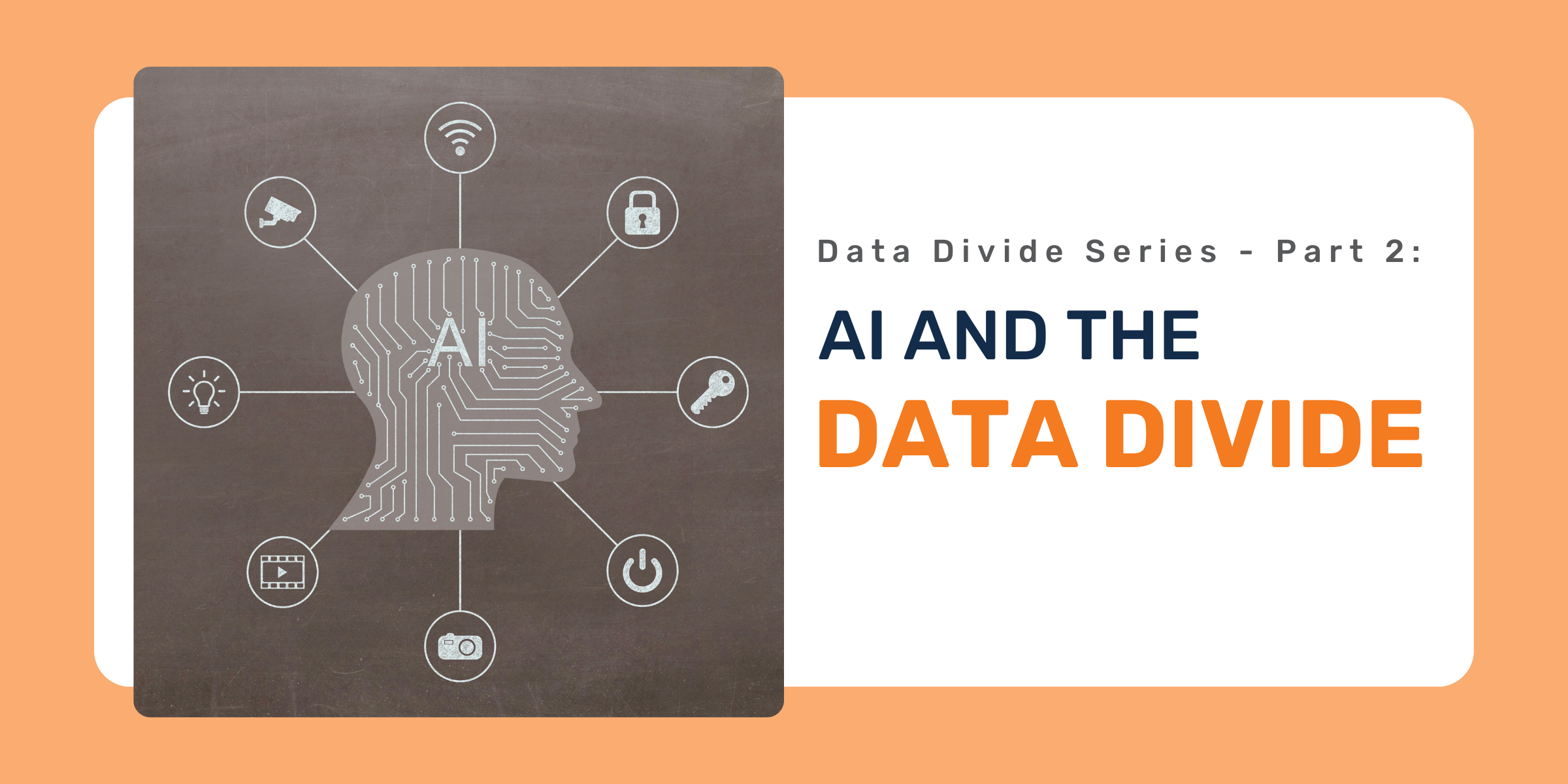
AI is not merely a buzzword or a passing trend; it has become an integral part of industries such as healthcare, finance, manufacturing, and more. Its capabilities to process, analyze, and interpret data at unprecedented speeds have paved the way for groundbreaking advancements. From diagnosing diseases with remarkable accuracy to optimizing supply chain logistics, AI is reshaping the way we live and work.
Yet, within the astounding rise of AI, a paradox looms large. It's a paradox that requires us to confront a challenging truth: while AI's prowess is hinged upon its insatiable hunger for data, its proliferation can potentially exacerbate a deeply ingrained divide - the Data Divide.
What is the Data Divide?
The Data Divide, as explored in the first article in this series, refers to the unequal distribution of data accessibility, quality, and utilization. It disproportionately impacts smaller organizations and underserved communities, constraining their ability to harness the transformative potential of data-driven technologies.
And here lies the conundrum: AI, which thrives on data as its lifeblood, can inadvertently perpetuate this divide, making it even wider and more challenging to bridge.
The Power and Promise of Artificial Intelligence
Artificial Intelligence, often referred to as the "new electricity" of the digital age, has ushered in a transformative era across diverse industries. Its impact is akin to a seismic shift, altering the very foundations of how we approach problems, make decisions, and drive innovation.
Analysis
Central to AI's remarkable capabilities is its proficiency in handling massive volumes of data. AI thrives on data like a detective relies on clues. It ingests, processes, and analyzes data with unprecedented speed and precision, unveiling patterns, correlations, and insights that elude human analysis.
AI's data prowess is especially evident in the field of data analytics. It can sift through terabytes of data in milliseconds, identifying trends and anomalies that might take human analysts weeks or even months to discover. This capacity for data-driven decision-making has far-reaching implications for businesses and organizations.
Automation
Automation is another cornerstone of AI's data-driven capabilities. AI-powered systems can automate mundane and repetitive tasks across industries, liberating human workers to focus on tasks that require creativity and critical thinking. In customer service, chatbots and virtual assistants use natural language processing to provide round-the-clock support. In manufacturing, robots execute intricate assembly tasks with precision, reducing error rates and improving efficiency.
Prediction
AI's ability to automate and streamline workflows extends to predictive analytics. By analyzing historical data, AI can make predictions about future events, such as equipment failures, customer behavior, or market trends. This predictive power empowers organizations to make proactive decisions and allocate resources more efficiently.
In essence, AI serves as the modern-day data whisperer, extracting valuable insights, optimizing operations, and enabling innovation across various sectors. However, as we delve deeper into the intricate relationship between AI and data, we begin to unravel the paradox of how AI, while being a data-driven marvel, can potentially exacerbate the Data Divide that restricts access to its transformative capabilities.
AI's Data Hunger
Artificial Intelligence is a voracious consumer of data, and its insatiable appetite is a driving force behind the growing Data Divide. To understand this paradox, we must delve into the immense data requirements of AI and how they perpetuate disparities in data accessibility and quality.
AI, particularly machine learning and deep learning models, thrives on high-quality data. These algorithms rely on data inputs to learn, make predictions, and adapt over time. The quality of the data they ingest directly impacts their performance and accuracy.
High-quality data is not just about having a large volume of information; it's also about having data that is accurate, diverse, and representative of the real-world scenarios the AI system will encounter. Clean, reliable data is the nourishment that AI models need to function optimally. However, the ability to access and curate such data is a privilege often enjoyed by larger organizations with extensive resources.
Training AI Models Requires Large and Diverse Datasets
Training AI models is akin to educating a bright student. They need exposure to a wide range of experiences and examples to develop a nuanced understanding of the world. AI models, particularly those based on machine learning, undergo extensive training processes where they analyze vast datasets to identify patterns, correlations, and associations.
The challenge arises when we consider the availability of such data, especially for smaller organizations and underserved communities. These entities often lack access to expansive and diverse datasets, hindering their ability to train AI models effectively. Consequently, they find themselves at a disadvantage in harnessing the full potential of AI for their specific needs
AI's data hunger, while driving innovation and progress, deepens the Data Divide. The quest for extensive and diverse datasets creates a chasm between those with access to abundant data resources and those who must make do with limited or biased datasets. Bridging this aspect of the Data Divide requires concerted efforts to democratize data collection, sharing, and curation, ensuring that AI's insatiable appetite for high-quality and diverse data can be satisfied across a broader spectrum of organizations and communities.
The Data Divide: A Barrier to AI Adoption
The Data Divide represents a paradox in the age of AI. At its core, the Data Divide encapsulates the disparities in data accessibility, quality, and utilization that exist among different entities, whether they are organizations, communities, or individuals.
Data Accessibility
Data accessibility, in this context, refers to the ability of various groups to acquire and harness data for their benefit. While data has become an abundant resource in our information-rich world, it is far from equally distributed. Some organizations have access to vast troves of data, while others grapple with data scarcity. This imbalance in data availability can be likened to a modern-day gold rush, where the fortunate few have access to abundant mines while others have to make do with limited resources.
Data Quality
Quality is the second dimension of the Data Divide, encompassing the accuracy, completeness, and reliability of data. Just as a treasure chest is worthless if filled with fool's gold, data loses its value when plagued by inaccuracies, omissions, or biases. The stark reality is that many organizations, particularly smaller ones and underserved communities, struggle with the quality of their data. Inaccurate or incomplete data can lead to erroneous AI predictions, flawed decision-making, and missed opportunities for innovation.
Data Utilization
However, data utilization stands as the third critical dimension of the Data Divide, and it is often the linchpin that determines whether data, regardless of its accessibility or quality, can truly empower AI adoption.
Data utilization represents the capacity of entities to effectively and efficiently employ data to drive decision-making, fuel innovation, and create value. It's the ability to turn raw data into actionable insights, and then, crucially, to act upon those insights. In this dimension, the divide manifests as a gap between those who possess the skills, tools, and infrastructure needed to extract value from data and those who struggle to do so.
The Dilemma of Smaller Entities
Smaller organizations and underserved communities, constrained by limited resources and expertise, often grapple with underutilized data. The cost of data acquisition, storage, and analysis can be prohibitive, leaving these organizations on the sidelines of the AI revolution. Their inability to access and leverage data-driven insights can hinder their competitiveness, limit their growth potential, and increase the risk of obsolescence in an increasingly data-centric business landscape.
Underserved communities face a similar predicament, albeit with broader social implications. Access to essential services, such as healthcare, education, and economic opportunities, is contingent on data-driven decision-making. However, when these communities are denied equitable access to data and the AI technologies that rely on it, they become trapped in a cycle of inequality. Health disparities persist, educational gaps widen, and economic opportunities remain elusive, all exacerbated by the Data Divide.
Conversely, larger organizations with robust data utilization capabilities are positioned to derive maximum benefit from their data assets. AI technologies can analyze vast datasets to reveal hidden patterns, automate tasks, and optimize operations. These organizations can harness data-driven insights to stay competitive, adapt swiftly to changing market dynamics, and fuel innovation.
In the age of AI, where data-driven innovation has the potential to revolutionize industries and improve lives, the Data Divide threatens to widen the chasm of inequality. Bridging this divide becomes not just a technological challenge but a moral imperative, as failing to do so perpetuates disparities that compromise the well-being and progress of society as a whole.
Closing Thoughts
The interplay between AI and the Data Divide is multifaceted and paradoxical. AI has the potential to democratize access to insights and innovation, but it also tends to favor those with abundant, high-quality data resources. This dynamic creates both opportunities and challenges:
-
Opportunities: AI can empower organizations to make data-driven decisions, automate processes, and gain a competitive edge. It has the potential to level the playing field and drive progress across various sectors.
-
Challenges: The Data Divide can hinder the adoption and effectiveness of AI for smaller entities, perpetuating disparities and limiting their ability to compete and innovate.
As we move forward in an AI-driven world, it's crucial to recognize the complex relationship between AI and data. We must strive for a future where AI benefits all, bridging the Data Divide rather than widening it further.
In closing, let us consider how we can collectively address these challenges. By advocating for equitable data access, quality, and utilization, we can harness the full potential of AI to create a more inclusive and prosperous future for everyone.
Bridge the Data Divide with TimeXtender!
Click here to get started with a FREE trial and try out all the capabilities you need to create a robust foundation for analytics and AI, without a large team or a complex stack of expensive tools!