3 min read
An Interview with TimeXtender Solution Specialist Taylor Hagin
Written by: TimeXtender - July 9, 2020
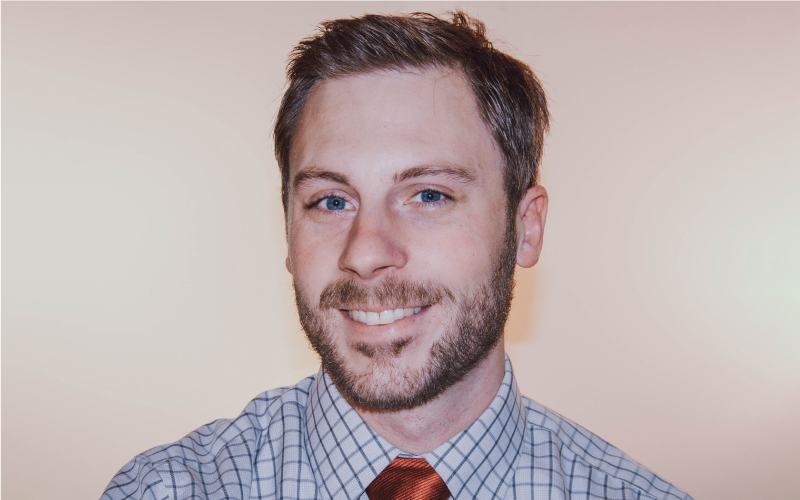
Q&A Takes a Look at Data Science and AI and Where Industry Might be Headed
Taylor Hagin is a Solution Specialist who recently joined the TimeXtender team. We sat down with Taylor and discussed his reasoning for joining TimeXtender, his background, and where he sees the industry moving to in the near future.
TimeXtender: You’re a fairly recent addition to the TimeXtender team. What piqued your interest about joining TimeXtender?
Taylor: TimeXtender has an amazing culture and value system. I found this really quite appealing. I also believe wholeheartedly that TimeXtender’s technology is the wave of the future. The platform is truly outstanding and leveraging the power of automation is so crucial for companies these days. Personally, career wise, I came from a consulting background and was interested in getting more on the side of offering a technology solution for business customers.
TimeXtender: As a Solution Specialist, you’re heavily involved with TimeXtender’s modern data estate technology. What would you say is unique about the technology platform?
Taylor: This is the only technology that I know of that solves so many headaches that are very common in data warehousing and data estate modernization. The technology truly is what its description implies: “a modern data estate.”
TimeXtender: What has been your area of expertise during your professional career?
Taylor: I worked diligently during my consulting career to try and meet the needs of customers. With that approach, I feel as though I was able to become a trusted advisor for them. Along with providing consulting expertise regarding data science initiatives, I also focused on delivering bottom-line business outcome and helping customers achieve pragmatic goals.
TimeXtender: Keeping with data science, where would you say this technology is headed in the near future?
Taylor: I see the data science space moving towards graph databases and also into NoSQL. I would also share that I believe “wise is greater than intelligent.” I would define smart as being reactive, logical, and measurement focused, whereas wise is proactive, emotional, and outcome based. For example, in the field of Natural Language Processing, many of the approaches to interpreting language (smart) is focused on the words themselves rather than the meaning of a text, or the impact that it may have (wise).
TimeXtender: What’s one misconception that people have about data science?
Taylor: When people in industry think about data science they often think that this needs to be done by a Ph.D. for it to be effective data science. People also have a misunderstanding that data science is this mythical aspect of data management that is really complicated and that needs to be advanced to be valuable. None of these cases are true.
TimeXtender: Ultimately, how does a company get more value out of data science?
Taylor: If a company understands the data science process, they know to focus more on outcomes than on the metrics. They should nurture a holistic view of their data strategy to better capture their data and to get better insights from their advanced analytics. They should also ensure security measures are in place. The better the data the better the understanding. Data should be accessible as needed when needed. Having an understanding of this can help stakeholders and data consumers get more from their data. It all starts with educating those involved with data.
TimeXtender: We hear a lot of talk about how hot AI is right now. You’ve also mentioned before that you don’t need AI for data science to be effective. Can you elaborate?
Taylor: AI is not as hot as people make it out to be. Many people don’t have a clear understanding what AI is and isn't. It’s just a broad term that is used to mean lots of different things to lots of different people. A vast majority of data science problems can be handled with a linear or logistic regression, applied correctly. AI is cool but doesn’t solve a broad set of business problems, as much as very specific problems. A balance of time, effort, and actionable insight to solve a business problem is key to driving value from a data science team.
TimeXtender: Final question, where do you see the data-management space in one year?
Taylor: Given COVID and its global ramifications, I think data management may become much more focused on data rights and transparency. I’d like to see more conversation about graph databases in this space. I’d also like to see more hybrid programs with Data Engineering (DE) focus (~80%) and some Data Science (DS) material (~20%), as the data analytics field needs more DE and analysts involved with deep learning (i.e. ETL + Analytics). The value of a resource to get to the right answer quickly is a competitive advantage, and largely hangs on the ability to get the right data, transformed in the right way. This is what a data scientist spends much of their time doing, and from what I’ve seen, the skills to do so are often learned on the job more than in industry curriculum.